Unlock Collaborative Robot Potential: Nonparametric Bayesian Learning for Multimodal Introspection

In the realm of robotics, collaborative robots (cobots) are gaining prominence as they seamlessly integrate into human workspaces, offering enhanced productivity and efficiency. However, to fully harness the potential of cobots, they require a deep understanding of their own state and capabilities, known as multimodal introspection.
4.5 out of 5
Language | : | English |
File size | : | 6631 KB |
Print length | : | 154 pages |
Challenges in Robot Introspection
Traditional robot introspection methods often rely on explicit, hand-crafted models that are tailored to specific tasks or environments. This approach faces significant limitations, as it becomes infeasible to manually encode the vast and diverse range of scenarios that robots may encounter in real-world applications.
Introducing Nonparametric Bayesian Learning
Nonparametric Bayesian learning offers a transformative solution to the challenges of robot introspection. This powerful statistical framework allows robots to infer their state and capabilities without relying on predefined models. Instead, it leverages Bayesian inference to construct a probabilistic distribution over the robot's parameters, based on observed data and prior knowledge.
Benefits of Nonparametric Bayesian Learning
- Adaptability: Nonparametric Bayesian learning enables robots to adapt to new environments and tasks without requiring extensive reprogramming.
- Uncertainty Quantification: It provides a measure of uncertainty associated with the robot's state estimates, enabling more informed decision-making.
- Robustness: Nonparametric Bayesian learning algorithms are robust to noise and outliers in the data, ensuring reliable introspection even in challenging environments.
Multimodal Introspection for Collaborative Robots
Nonparametric Bayesian learning is particularly well-suited for multimodal introspection in collaborative robots. By combining data from multiple sensors, such as cameras, force sensors, and encoders, robots can gain a comprehensive understanding of their surroundings, their own physical capabilities, and the intentions of human collaborators.
This multimodal introspection enables cobots to:
- Enhanced Situational Awareness: Understand the environment and predict the actions of human collaborators, improving coordination and safety.
- Self-Calibration: Continuously adapt their internal models to compensate for changes in the environment or their own hardware.
- Human-Robot Collaboration: Communicate their state and intentions to human collaborators, facilitating seamless and efficient teamwork.
Applications in Robotics
The applications of nonparametric Bayesian learning for collaborative robot multimodal introspection are far-reaching. It has the potential to revolutionize various robotics domains, including:
- Industrial Automation: Cobots in manufacturing environments can continuously learn and adapt to changing production lines.
- Healthcare: Surgical robots can gain a detailed understanding of patient anatomy and surgical instruments, leading to safer and more precise procedures.
- Autonomous Vehicles: Self-driving cars can improve their perception and decision-making by continuously learning about their surroundings and their own dynamics.
Nonparametric Bayesian learning for collaborative robot multimodal introspection represents a paradigm shift in robotics. By enabling robots to gain a deep understanding of their own state and capabilities, it unlocks new levels of autonomy, adaptability, and human-robot collaboration. As the field of robotics continues to evolve, nonparametric Bayesian learning will undoubtedly play a pivotal role in shaping the future of intelligent machines.
References
- Atkeson CG, Schaal S. Robot Learning from Demonstration. In: Handbook of Robotics. Springer; 2008.
- Calinon S, Billard A. Incremental Learning of Inverse Kinematics with a Dynamical Movement Primitive. In: Proceedings of the IEEE International Conference on Robotics and Automation. IEEE; 2007.
- Deisenroth MP, Faisal AA, Ong CS. Mathematics for Machine Learning. Cambridge University Press; 2020.
4.5 out of 5
Language | : | English |
File size | : | 6631 KB |
Print length | : | 154 pages |
Do you want to contribute by writing guest posts on this blog?
Please contact us and send us a resume of previous articles that you have written.
Book
Novel
Page
Chapter
Text
Story
Genre
Reader
Library
Paperback
E-book
Magazine
Newspaper
Paragraph
Sentence
Bookmark
Shelf
Glossary
Bibliography
Foreword
Preface
Synopsis
Annotation
Footnote
Manuscript
Scroll
Codex
Tome
Bestseller
Classics
Library card
Narrative
Biography
Autobiography
Memoir
Reference
Encyclopedia
Suzanne Lambert
Beryl Allen
Eugene Franklin Clark
10th Edition Kindle Edition
Adrienne Maciain Phd
Don E Wilson
Gareth C Sampson
William M Fowler
Hitesh Tikamchand Jain
Josephine Humphreys
Emma Griffiths
Giovanbattista Tusa
Kelly Peasgood
Thomas C Foster
J Paul Guyer
Patrick Foote
Mark Eagle
Cain Quillman
Jack L Daniel
Joe Mcnally
Light bulbAdvertise smarter! Our strategic ad space ensures maximum exposure. Reserve your spot today!
- William FaulknerFollow ·14.3k
- Will WardFollow ·15.7k
- Bradley DixonFollow ·5.9k
- Jon ReedFollow ·19.6k
- Ralph EllisonFollow ·6k
- Brett SimmonsFollow ·17.3k
- Shannon SimmonsFollow ·18.2k
- Darnell MitchellFollow ·8.7k
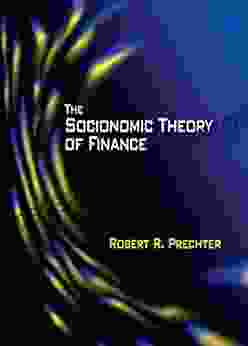

Unlock Your Financial Future: Discover the Transformative...
In a tumultuous and ever-evolving financial...
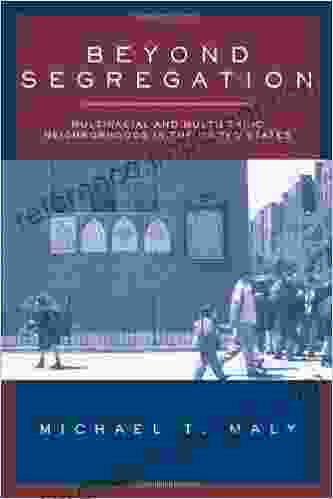

Beyond Segregation: Multiracial and Multiethnic...
The United States has a long history of...
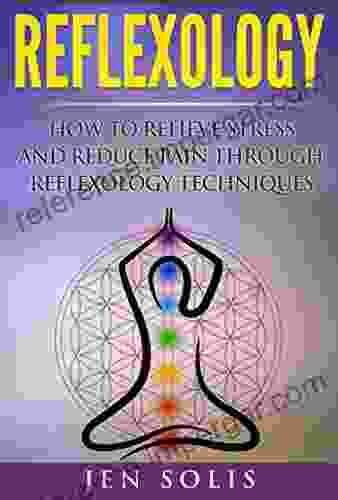

Unlock the Secrets of Reflexology: A Journey to Stress...
Explore the...
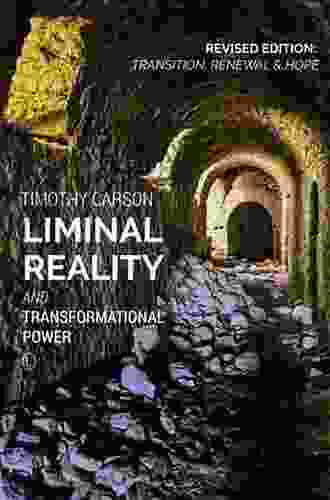

Liminal Reality and Transformational Power: Exploring the...
Life is a constant...
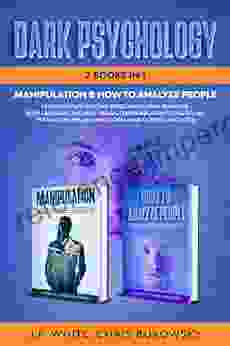

Unlock the Secrets of Human Behavior: A Comprehensive...
Have you ever wondered...
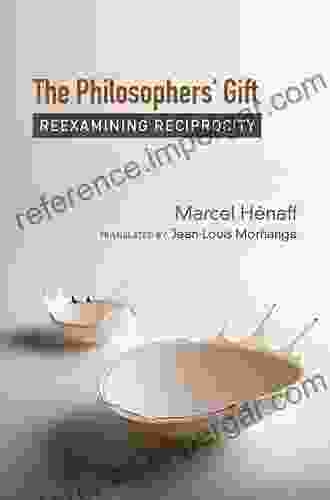

The Philosopher's Gift: Reexamining Reciprocity
The concept of reciprocity, the idea that...
4.5 out of 5
Language | : | English |
File size | : | 6631 KB |
Print length | : | 154 pages |